Impact of AI on Data Management: A Comprehensive Guide
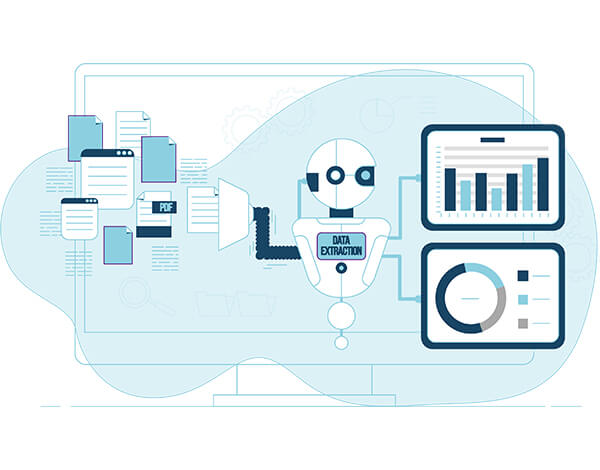
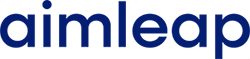
Estimated Reading Time 9 min
AIMLEAP Automation Works Startups | Digital | Innovation | Transformation
Streamlining data management is crucial for precision in predictive analytics. This process, traditionally labor-intensive, includes various stages such as data acquisition, purification, organization, and categorization, all preceding the analysis phase.
Integrating artificial intelligence into data management promises to refine these steps significantly. AI is projected to enhance worker efficiency by 40% by 2035 (claims this report). Leveraging AI for automating data processing tasks – including cleansing, sorting, analyzing, and spotting irregularities – not only increases productivity but also empowers data analysts. They can then dedicate more time to interpreting analytical outcomes, facilitating informed, data-centric decision-making in your organization.
While promising results are evident, let us dive deeper into the impact of AI in data management.
Understanding AI and data management
The first step towards understanding the impact of AI in data management lies is understanding what AI and data management are. These definitions will help:
- Artificial intelligence, abbreviated as AI, focuses on creating machines capable of thinking and learning like humans. It involves programming computers to process information, make decisions, and solve problems, much like your brain does. This technology powers things such as voice assistants, recommendation systems, and self-driving cars, making them smarter and more efficient. Essentially, AI is about making machines that can perform tasks that would normally require human intelligence.
- Data management is the method of collecting, storing, and using data securely, efficiently, and cost-effectively. Think of it as organizing and maintaining a library of information. This involves processes such as acquiring data, validating its accuracy, storing it safely, ensuring it’s accessible when needed, and protecting it from unauthorized access.
- Integrating AI into data management automates routine tasks, enhances data accuracy, and offers advanced analytics. It enables efficient handling of large data volumes, providing businesses with insights for better decision-making. AI-driven data management also aids in predictive analytics, improves data security, and personalizes customer experiences, transforming data handling into a more efficient, proactive process, crucial for informed business strategies.
10 ways AI transforms data management
AI transforms data management in several significant ways. It changes how data is managed, simplifies operations, enhances security, and provides deeper insights and foresight. Let us explore some benefits of AI in data management in detail.
1. Automates data entry and processing
AI in data management uses machine learning and natural language processing (NLP) to automate data entry and processing. Machine learning identifies patterns, enhancing data input accuracy over time. NLP interprets human language for the automated processing of written or spoken data. For example, in retail, AI employs optical character recognition (OCR) to digitize text from customer feedback surveys. It then sorts this feedback by sentiment, updating customer profiles. This reduces manual effort and improves accuracy in data handling.
2. Automates data categorization and tagging
Advanced AI algorithms automatically sort and classify large volumes of data. These algorithms learn from existing data, identifying patterns and themes to organize data into relevant categories. For instance, in an online news portal, AI can categorize articles into topics like sports, politics, or entertainment based on their content and keywords. This not only streamlines the process of managing and retrieving data but also significantly improves the precision and efficiency of data categorization, making it a valuable tool for businesses dealing with extensive data sets.
3. Improves data quality with cleansing and validation
AI identifies and fixes inaccuracies, fills missing values, and removes duplicate entries, thereby ensuring data integrity. For validation, AI uses pattern recognition to verify data against predefined rules or historical data patterns. For example, in a customer database, AI can detect and correct erroneous entries like misspelled names or invalid phone numbers, and validate new entries to maintain a high-quality, reliable database, crucial for effective business decision-making.
4. Enforces regulatory compliance
AI algorithms scan data sets for compliance with regulations like GDPR or HIPAA, identifying and flagging any non-compliant data. AI systems can also be programmed to automatically update data handling processes in response to changes in legislation. For example, in healthcare, AI can ensure patient data is managed in compliance with privacy laws by automatically anonymizing personal information and restricting access as per regulatory requirements, thus maintaining legal compliance and safeguarding sensitive data.
5. Supports predictive analytics
AI analyzes vast datasets to forecast future trends and behaviors. It employs machine learning models to identify patterns and correlations within historical data, enabling accurate predictions about future events or outcomes. For instance, in retail, AI can analyze past sales data, customer preferences, and market trends to predict future product demands. This predictive insight helps businesses in inventory planning, marketing strategies, and enhancing customer experiences by anticipating their needs. This capability of AI to provide foresight based on data-driven predictions is invaluable for strategic planning and decision-making in various industries.
6. Detects data security issues and anomalies
AI enhances data security by detecting anomalies and potential threats. It continuously monitors data for unusual patterns or activities, indicative of security breaches or internal threats. For instance, in financial institutions, AI can identify irregularities in transaction patterns, flagging potentially fraudulent activities. It does this by comparing current transactions against historical data and known fraud patterns. By promptly detecting these anomalies, AI plays a crucial role in preemptive threat identification, thereby safeguarding sensitive data and mitigating risks associated with data breaches or financial fraud. This proactive approach is essential for maintaining robust data security.
7. Enhances data analytics
AI significantly enhances analytics in data management by enabling deeper and more complex analysis of large data sets. It uncovers hidden patterns, trends, and insights that might be too subtle or complex for traditional analysis methods. For example, in e-commerce, AI can analyze customer purchase history, browsing behavior, and demographic data to provide personalized product recommendations.
8. Assists in decision making
AI aids decision-making in data management by providing actionable insights derived from complex data analysis. It processes vast amounts of data to identify trends, patterns, and correlations, offering a more informed basis for decisions. For example, in supply chain management, AI can analyze market trends, historical sales data, and inventory levels to recommend optimal stock quantities. By turning data into strategic insights, AI empowers businesses to make data-driven decisions, enhancing their responsiveness and competitiveness in the market.
9. Facilitates data migration
AI streamlines the transfer of data from one system to another by mapping data fields between different formats and systems. AI can also identify and rectify inconsistencies or errors in the data during migration. For example, when a company moves its customer database to a new CRM system, AI can automate the mapping of customer records, maintaining data integrity and consistency. This
reduces the time and resources typically required for data migration, while minimizing the risk of data loss or corruption, thereby ensuring a smooth transition.
10. Supports continuous improvement
AI constantly learns and adapts from data interactions. With machine learning algorithms, it evolves over time, enhancing data processing, analysis, and decision-making. For instance, in customer relationship management, AI can track the effectiveness of different communication strategies and customer interactions. Based on this data, it refines its understanding of customer preferences and behavior, leading to more targeted and effective marketing strategies. This iterative learning process ensures that data management systems remain dynamic and increasingly efficient, continually evolving to meet the changing needs and challenges of the business environment.
5 challenges of AI in data management
While the list of benefits of AI in data management is long, there are some challenges too. Learning about these challenges is crucial before integrating AI into data management processes.
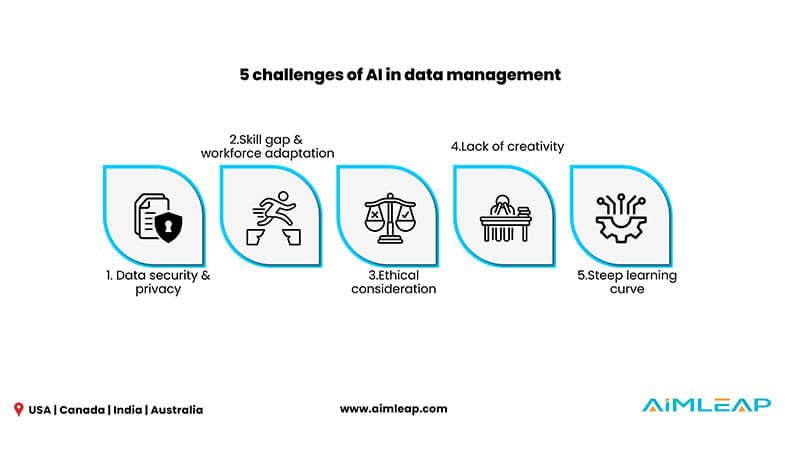
- Data security and privacy: AI processes vast amounts of data, including sensitive information. This increases the risk of data breaches and misuse. Ensuring the confidentiality, integrity, and availability of data, while complying with privacy regulations like GDPR, thus becomes crucial. Balancing the potential of AI with robust security and privacy protections remains a key concern for organizations.
- Skill gap and workforce adaptation: AI technologies are continuously advancing which increases the need for skilled professionals who understand AI and data science. This creates a gap between available expertise and the demands of AI-driven systems. Additionally, adapting the existing workforce to work alongside AI, through training and development, is essential yet challenging for many organizations.
- Ethical consideration: Ethical considerations in AI-driven data management involve addressing biases in algorithms, ensuring fairness, and maintaining transparency in AI decision-making processes. . Ensuring these systems are designed and operated ethically, with accountability for decisions made by AI, is a significant challenge, requiring ongoing attention to ethical principles in AI development and deployment.
- Lack of creativity: AI, primarily functioning on pre-existing data and set algorithms, lacks the ability to think abstractly or innovate beyond its programming. This limitation means AI can’t replicate the creative problem-solving and innovative thinking that human minds offer, potentially leading to less dynamic solutions and missed opportunities for novel approaches in data management.
- Steep learning curve: Implementing and effectively using AI requires a deep understanding of complex algorithms and data science principles. For many organizations, this means investing in specialized training and development, which can be time-consuming and costly. This barrier often makes it difficult for businesses to fully leverage AI’s potential in managing and analyzing their data.
AI in data management: What are big brands doing?
Big brands are innovatively using AI in data management to boost efficiency, gain valuable insights, and stay ahead in the market. Here are four key examples to help you understand how these brands are implementing AI, offering inspiration for your strategies:
1. Google BigQuery
Google BigQuery is a serverless data warehouse that integrates AI for advanced data management. It facilitates large-scale data analysis with machine learning capabilities, allowing users to gain insights without managing infrastructure. BigQuery’s AI integration helps automate data insights, making data analysis more accessible and efficient. It enables predictive analytics and offers tools for machine learning directly within the data warehouse, streamlining the process of turning vast data sets into actionable intelligence for businesses.
2. IBM Watson Data Studio
IBM leverages AI in data management through Watson Studio, part of Cloud Pak for Data as a Service (DaaS). Watson Studio offers an environment and tools for collaborative data work, supporting tasks like data analysis, cleansing, and machine learning model creation. It emphasizes collaboration, with resources like data assets and operational assets organized in project workspaces. This setup streamlines data management and enhances AI-driven analytics, allowing teams to derive insights more effectively. Watson Studio integrates with services like Watson Knowledge Catalog for data governance, ensuring comprehensive management and utilization of data.
3. Microsoft Azure Data Factory
Microsoft Azure Data Factory provides a fully managed, serverless data integration service that facilitates the creation, scheduling, and orchestration of data flows. Azure Data Factory enables the combination of data from various sources, transforming it with computing services like Azure HDInsight and Azure Machine Learning. This integration allows for efficient data processing and movement, enhancing data-driven decision-making and enabling complex data analytics workflows in a scalable and secure environment.
4. Salesforce Einstein Prediction
Microsoft Azure Data Factory provides a fully managed, serverless data integration service that facilitates the creation, scheduling, and orchestration of data flows. Azure Data Factory enables the combination of data from various sources, transforming it with computing services like Azure HDInsight and Azure Machine Learning. This integration allows for efficient data processing and movement, enhancing data-driven decision-making and enabling complex data analytics workflows in a scalable and secure environment.
Next steps to take to implement AI in data management
To successfully implement AI in data management, consider these five key steps:
Step 1: Define AI goals and objectives
As a first step, identify specific outcomes you want to achieve with AI, such as enhancing data accuracy, improving efficiency, or gaining deeper insights. Clear goals guide the selection of appropriate AI tools and strategies, ensuring that the implementation is aligned with the organization’s broader business objectives. This clarity helps in measuring success and provides a focused direction for the entire AI integration process.
Step 2: Assess the current data management landscape
After identifying the goals, thoroughly review the existing data infrastructure and workflows. It’s important to understand the current state of data storage, processing capabilities, and any existing analytics tools. This evaluation helps identify areas where AI can bring improvements and ensures that the AI solutions chosen are compatible with the existing system. This step is vital for a smooth integration of AI, enabling it to effectively enhance data management processes.
Step 3: Select the appropriate AI solution
Now, move on to choosing AI technologies and tools that align with the defined objectives and current data landscape. This selection is based on factors like compatibility with existing infrastructure, ability to meet specific data analysis needs, scalability, ease of integration, and cost-effectiveness. The right AI solution should enhance data processing, analysis, and decision-making, tailored to the organization’s unique requirements and challenges in data management. This step is crucial for ensuring that the AI implementation is effective and delivers the desired outcomes.
Step 4: Prepare data for AI integration
Once you have the right AI technology at hand, start focusing on making your data suitable for AI use. This involves cleaning, structuring, and normalizing data to ensure its quality and reliability. It’s about removing inaccuracies, inconsistencies, and redundancies to create a robust dataset. Properly prepared data is critical for training accurate AI models. This process also includes categorizing and tagging data appropriately, which is essential for effective analysis and insights generation by the AI system.
Step 5: Integrate AI into data management processes
As the fifth and final step, embed the selected AI solutions into existing data workflows. This step is about ensuring the AI tools work seamlessly with current data systems, automating tasks like data entry, analysis, and reporting. It requires configuring the AI to interact effectively with the data infrastructure, and may involve customizing the AI algorithms to specific data-related tasks. Successful integration is marked by AI enhancing the efficiency, accuracy, and insights of data management processes, ultimately supporting better business decision-making.
Conclusion
AI’s impact on data management is transformative. This comprehensive guide has explored AI’s role in enhancing data efficiency, accuracy, and insights, while also addressing the challenges and ethical considerations. We have outlined key steps for successful AI integration and highlighted how leading brands are effectively leveraging AI for improved data management. As technology evolves, its role in data management will continue to grow, offering businesses innovative ways to harness their data for strategic advantage. Embracing AI in data management is not just a trend; it’s a pathway to future-proofing business operations.
Frequently asked questions
1. Can AI affect data management jobs?
AI can significantly affect data management jobs by automating routine tasks, leading to a shift in job roles towards more strategic, analytical, and creative tasks. Professionals may need to upskill to navigate the AI-driven data management landscape, focusing on AI oversight, data interpretation, and strategic decision-making.
2. What are the common applications of AI in data management?
Common applications of AI in data management include automating data cleaning and processing, enhancing data quality and accuracy, enabling predictive analytics for future trends, optimizing data storage and retrieval processes, and ensuring data security through intelligent threat detection and response mechanisms. These applications streamline operations and drive more informed decision-making.
3. What are the challenges of implementing AI in data management?
Implementing AI in data management presents challenges such as ensuring data quality and integrity, managing data privacy and security concerns, addressing the skills gap among employees to work with AI technologies, and integrating AI systems with existing IT infrastructure. Additionally, the cost of AI implementation and ongoing maintenance can be significant. These hurdles require careful planning and resource allocation to overcome.
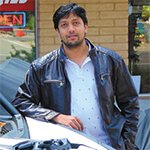
Jyothish Chief Data Officer
A visionary operations leader with over 14+ years of diverse industry experience in managing projects and teams across IT, automobile, aviation, and semiconductor product companies. Passionate about driving innovation and fostering collaborative teamwork and helping others achieve their goals.
Certified scuba diver, avid biker, and globe-trotter, he finds inspiration in exploring new horizons both in work and life. Through his impactful writing, he continues to inspire.
Related Articles
APISCRAPY – Web & App Data Scraping
Web & app scraping and automation platform that converts any web data into ready-to-use data API
PRICE SCRAPY – Real-Time Price Tracking
Track prices for products or services on multiple online platforms and make informed pricing decisions based on market trends and competitor pricing strategies
APIKART – Marketplace for Data API
Unleashing the Power of APIs: Discovering the Best Solutions for Your Business through Our API Marketplace